AI that identifies pixelated & blurred faces easy for hackers to exploit - researchers
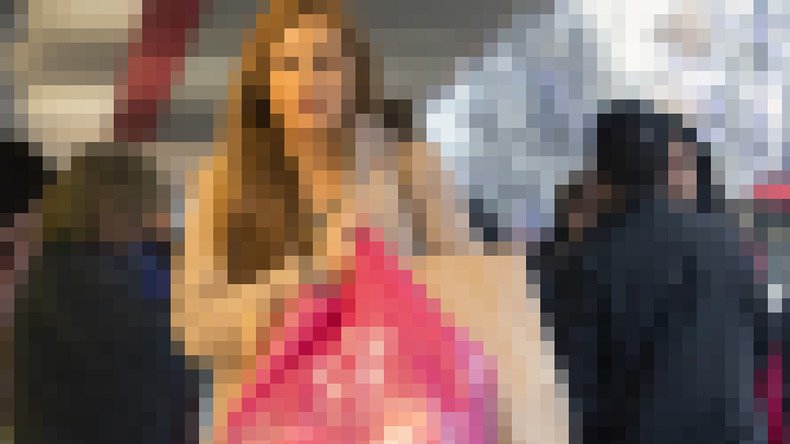
Security researchers are warning that commonly used visual redaction methods are no match for computer software which can identify blurred & pixelated images, unrecognizable to the human eye.
Researchers at Cornell University and University of Texas at Austin trained artificial neural networks to successfully identify faces, objects and handwritten digits even if the images were protected by obfuscation techniques such as blurring, mosaicing or P3 - a type of JPEG encryption.
What’s perhaps most worrying, however, is that they didn’t develop new advanced programming technology to do this but instead adapted mainstream machine learning methods to train the computer with a sample set of data.
“The techniques we’re using in this paper are very standard in image recognition, which is a disturbing thought,” Vitaly Shmatikov, one of the authors from Cornell Tech told Wired.
He warned that it would be possible for someone with rudimentary technical knowledge to carry out such an attack as there are online tutorials for those wishing to learn the machine learning methods used in the research.
Woah. Someone other than me is tweeting about my work! https://t.co/831bWgVEWf
— Richard McPherson (@rfmcpherson) September 6, 2016
The research raises concerns over privacy implications as pixelation is often used by the media to protect someone’s identity, for example a victim or a child or to censor graphic images.
Additionally obfuscation methods are used to blur out private and sensitive information such as license registration plates or parts of confidential documents.
These techniques partially remove sensitive information in order to make specific elements unrecognisable but retain the image's basic structure and appearance and allow conventional storage, compression, and processing.
While these techniques have successfully been used for years to mask parts of images from the human eye they are not so robust at being disguised from computer software.
READ MORE:Flash before your eyes: Hacking technology for law enforcement revealed
“We’re using this off-the-shelf, poor man’s approach,” Shmatikov told Quartz.
“Just take a bunch of training data, throw some neural networks on it, throw standard image recognition algorithms on it, and even with this approach… we can obtain pretty good results.”
The team tested their technique on YouTube’s blur tool using the pixelation technique, which can be used in Photoshop and other common editing programs and on Privacy Preserving Photo Sharing (P3) - a process that encrypts identifying data in JPEG photos so humans can’t see the overall image but maintains other data in the file.
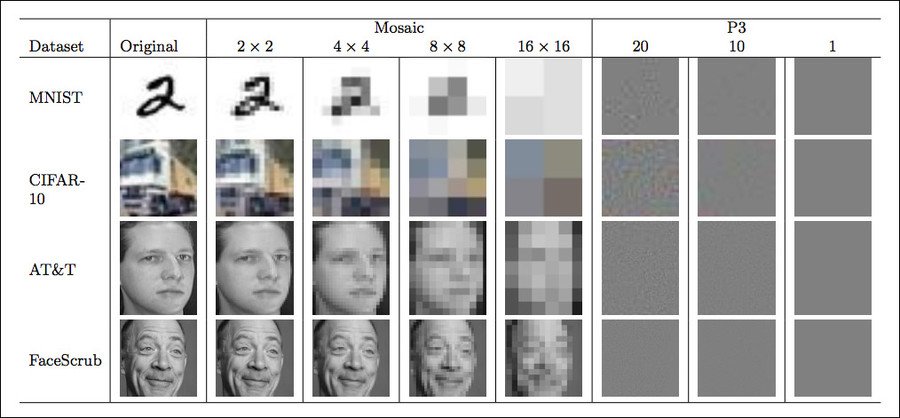
They first trained the computer to recognise objects in untouched images and then trained them to interpret blurred or pixelated images based on their knowledge of the originals.
Later they presented the neural networks with new obfuscated images. Success rates were greater than 80 and 90 percent in some cases.
The mosaiced images with the most intense pixelation had the lowest success rates but still ranged between 50 and 75 percent.
The researchers noted that the accuracy was impressive for such a simple network on a large dataset and shows that even simple methods can defeat current image masking techniques.
AI is everyone's new friend on Facebook, understanding posts & interfering in communication https://t.co/IyZsh957YXpic.twitter.com/OycoxR1Lfn
— RT (@RT_com) June 2, 2016
This technique can not restore the image completely however, but can retain enough information to allow “accurate reconstruction”.
Researchers say it is important that the designers of privacy protection technologies for visual data use state-of-the-art image recognition algorithms to establish how much information can be re-constructed.
“As the power of machine learning grows, this tradeoff will shift in favor of the adversaries,” the study noted.
Full encryption is not the answer, according to the team as, while it blocks all forms of image recognition, it comes at a cost of destroying all ability to use the image.
The key is developing privacy protection technologies that can protect faces in photos and videos while preserving the news value of these images, the research concluded.